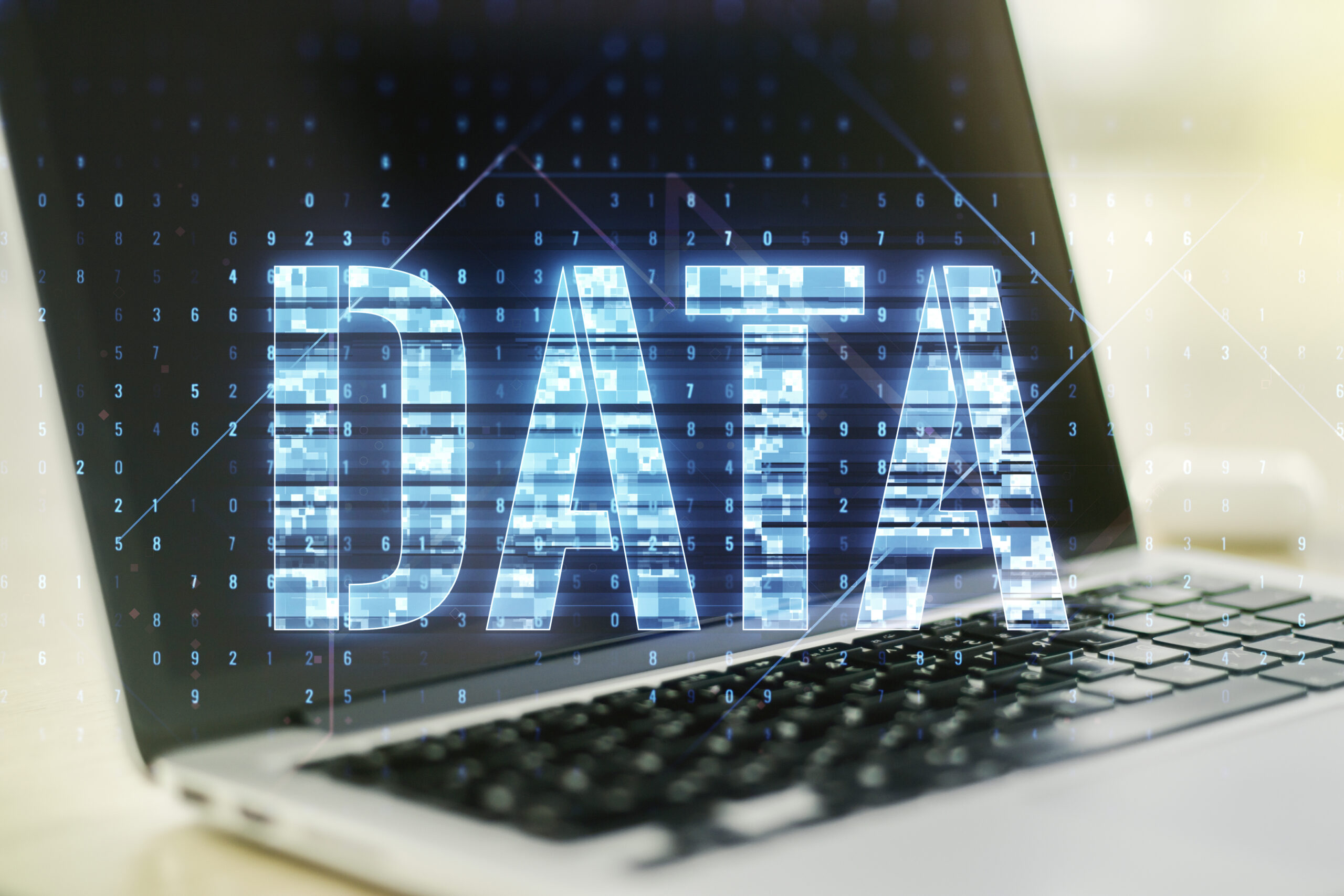
What is synthetic test data?
Synthetic test data is information that’s manufactured rather than generated by real-world events. Rather than compiling real-world records, synthetic datasets are collected using computer programs. Synthetic datasets are made to be flexible and long-lasting enough to help train machine-learning models.
Using practical synthetic data generation, companies can get training data much faster and cheaper than if they had to collect it from the real world, which is time-consuming. It is much easier to artificially generate 100,000 images of an object on an assembly line than it is to collect those images manually.
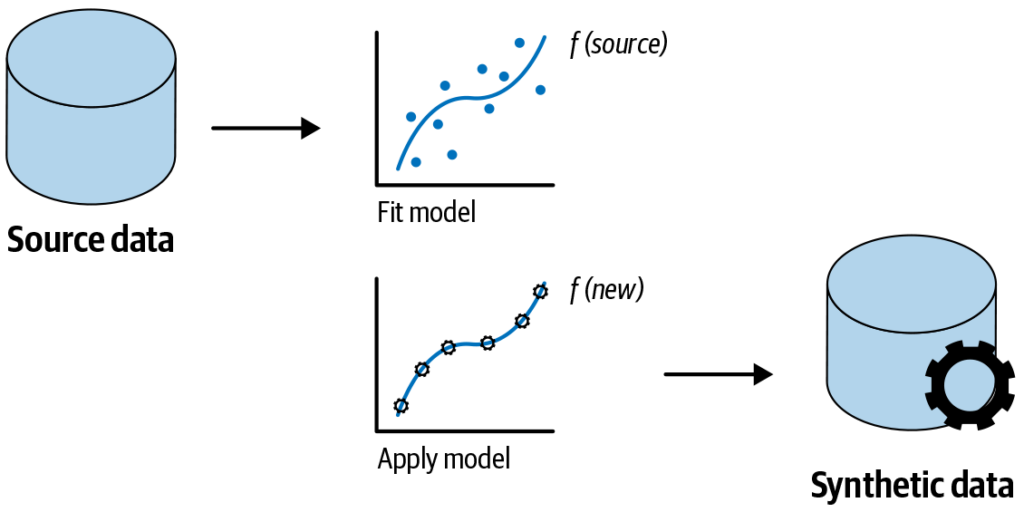
Why is practical synthetic data generation needed?
Various advantages come along with practical synthetic data generation. These include, but are not limited to, the following:
- Easing restrictions when using sensitive or regulated data
- Adjusting the data requirements to specific circumstances
- Producing datasets for DevOps teams to use for software testing and quality assurance.
Large data sets are often challenging for businesses to get on time and train an effective model. By using synthetic data, scientists and businesses can get past these barriers. They will also be able to create trustworthy machine-learning models with synthetic data.
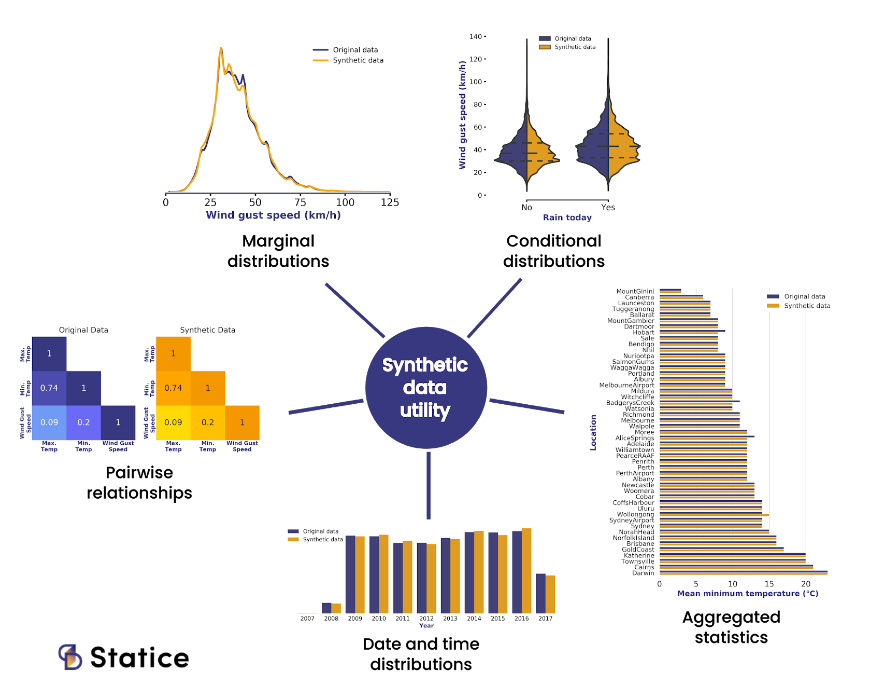
Use cases for synthetic test data
Synthetic data is beneficial to various machine-learning activities. Thus, synthetic data proves to be helpful for a broad range of applications, such as:
- Security,
- Robotics,
- Fraud prevention,
- And self-driving cars.
These are a few industries that often take advantage of synthetic data.
One of the first use cases for synthetic data was self-driving cars. Autonomous cars and synthetic data fit together exceptionally well. It would be impossible to get driving data from the real world for situations that an autonomous vehicle (AV) might face on the road.
So instead, AV companies developed sophisticated simulation engines. These engines gathered the needed data and showed AI systems the “long tail” of driving situations.
These simulated worlds generate dozens or millions of driving scenarios. Here are a few examples of data extracted and proposed using synthetic programs:
- Modifying automobile positions,
- Adding or removing pedestrians,
- Varying vehicle speeds, ETC.
Various autonomous vehicle players have invested in synthetic data, leaving it to act as a core part of their technology stack: including Waymo, Cruise, Auror, and Zoox.
Besides self-driving cars, there are plenty of other systems that incorporate synthetic data.
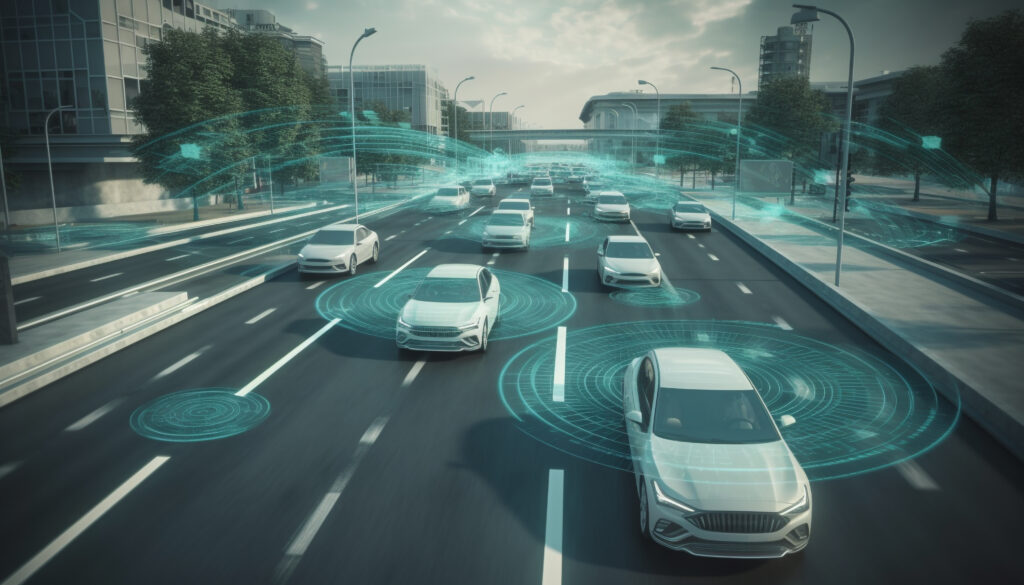
Use cases that have helped bring synthetic data to light
- Surveillance systems: Instead of collecting and labeling a lot of training data, which is more complex, synthetic data can be used to make training data for image recognition systems.
- Robotics systems: Robotic companies can test and design systems thanks to synthetic data instead of teaching and creating systems using conventional training techniques.
- Fraud protection: Synthetic data improves fraud prevention systems by providing new fraud detection techniques. These systems are enhanced with training and testing data that is continuously updated.
- The healthcare field: Synthetic data in the healthcare industry can create accurate health classifiers. Individual privacy remains protected since the data is not based on actual people.
- The healthcare field: Synthetic data in the healthcare industry can create accurate health classifiers. Individual privacy remains protected since the data is not based on actual people.
Risks of using synthetic data
While using synthetic test data has numerous benefits, there are also several drawbacks.
Synthetic test data often lacks outliers. In most cases, they’re removed from training datasets due to the natural appearance of data. However, their presence helps to develop machine learning models that are dependable.
The accuracy of synthetic data also tends to vary. Since the input, or “seed,” is often used to produce synthetic data, the quality of the output data may depend on the quality of the original data. The then-created data may reinforce any bias present.
Thus, synthetic data will require some output/quality control. The data will have to undergo proofreading and fact-checking, ensuring that the original data is legitimate.