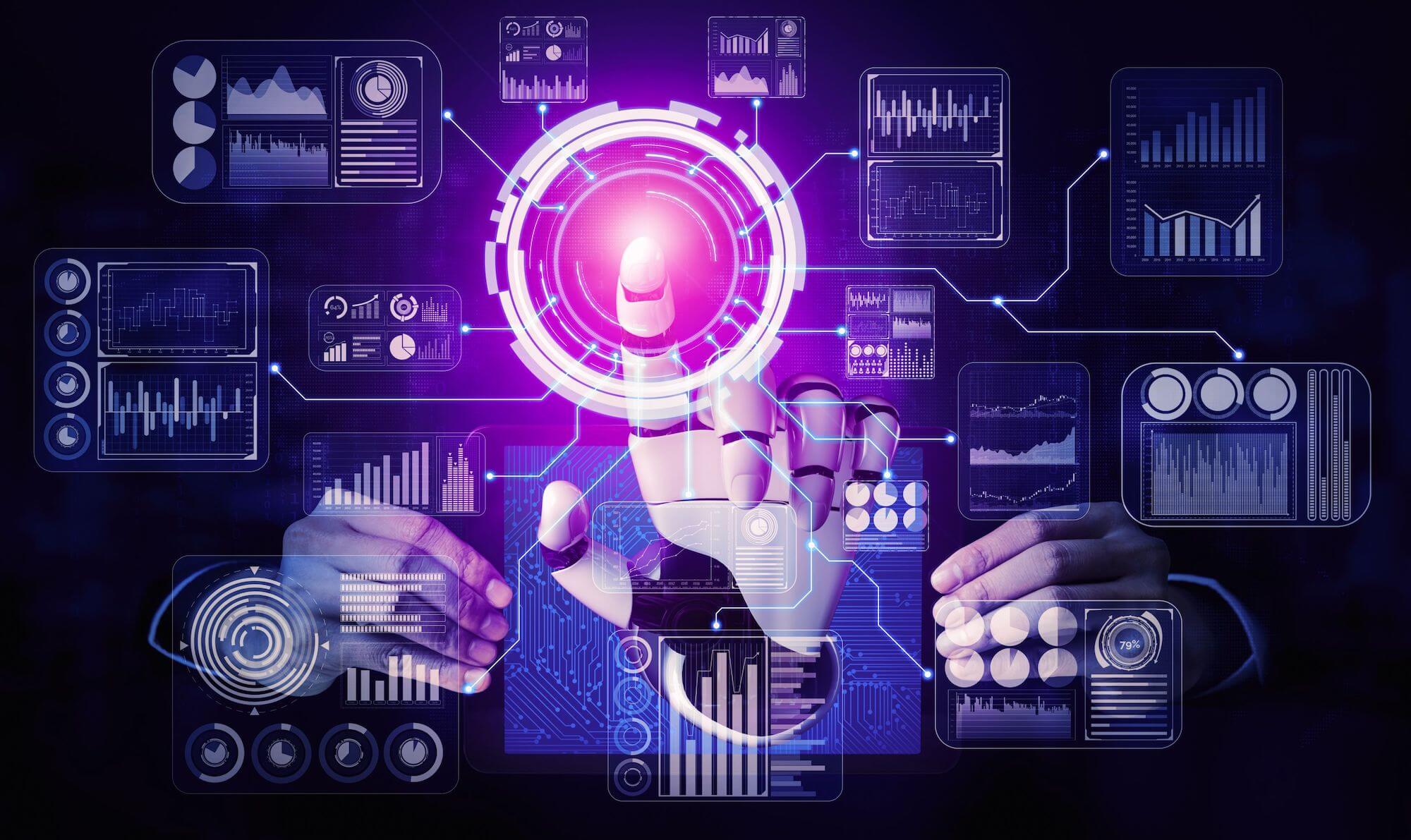
Ever since the launch of Chat GPT almost a year ago, the AI arena has been abuzz with conversations about the transformative power, and the economic potential, of generative AI. Yet, we have barely scratched the surface.
Beyond generative AI’s initial applications in creating text or images lies a vast territory of unexplored use cases. Our use case database is constantly updated with new generative AI applications across all industries, including manufacturing, telecommunications, automotive, banking, and beyond. But, as we dive into this brave new world, we need to tread carefully. Just like any other groundbreaking tech, there are hurdles to overcome and challenges to address. The way forward demands that we embrace generative AI ethics to guide its evolution and ensure sustainable development.
In this blog, we delve into the economic opportunities, emerging trends, and significant challenges that shape the landscape of generative AI, with a focal point on prioritizing ethical dimensions for its responsible evolution.
Let’s dive in.
What is Generative AI Really?
Unless you’ve been living off the grid for the past year, you already know something about generative AI. But to make sure we’re all on the same page, here’s a quick recap:
Generative AI refers to a category of artificial intelligence models that are designed to generate new data or content based on the patterns and structures learned from a given dataset. These models generate outputs like text, images, music, or even videos by mimicking the style, context, or other characteristics present in the training data.
How Does Generative AI Work?
One of the most well-known examples of generative AI models is the Generative Adversarial Network (GAN), which consists of two neural networks – a generator and a discriminator – that work together in a competitive process.
The generator creates new samples, while the discriminator evaluates the quality of these samples by determining whether they are real (from the training dataset) or fake (generated by the generator). Over time, the generator improves its ability to create realistic samples by learning from the feedback provided by the discriminator.
Another popular example is the Transformer architecture, which is used in natural language processing tasks, such as text generation or translation. OpenAI’s GPT (Generative Pre-trained Transformer) models are some examples that fall in this category. They are trained on massive amounts of text data and can generate human-like text based on given prompts while also being able to answer questions, summarize text, and complete sentences, among other tasks.
As you can imagine, the economic potential of generative AI is huge. The generative AI market is already exploding across all industries including art, music, marketing, finance, healthcare, and more. Its capabilities range from generating realistic images, composing music, creating personalized content, and designing new products to predicting financial trends, detecting fraud, and optimizing complex systems.
The real question is who defines generative AI ethics?
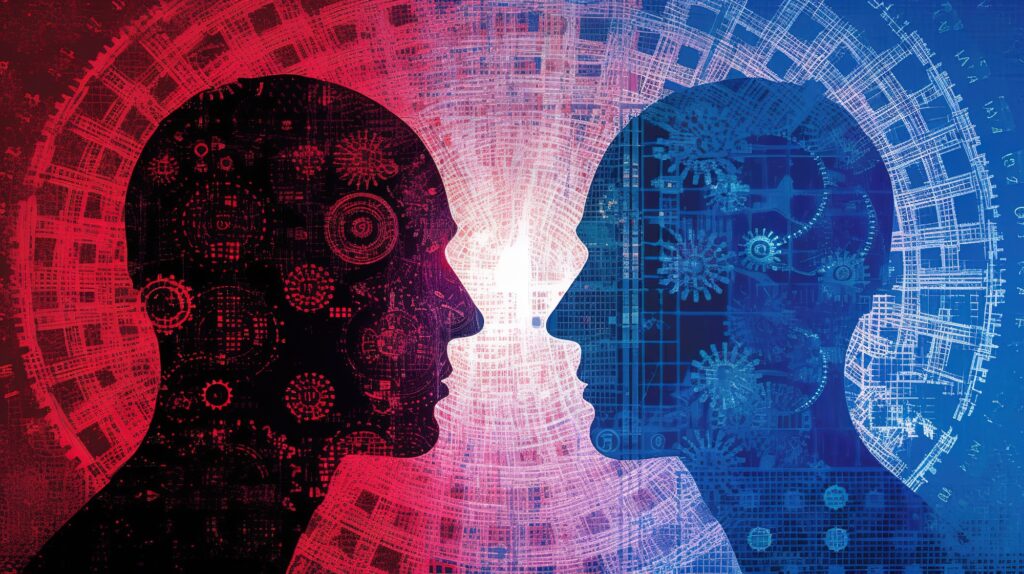
Generative AI Ethics
As with any other AI, generative AI poses risks to data privacy, security, and the workforce. It also has its own unique set of challenges. Let’s look at a few:
Misinformation
Perhaps the gravest threat posed by generative AI is the spread (whether malicious or accidental) of misinformation.
As this technology advances in sophistication, it is becoming more difficult to distinguish fake content from real content. This poses a real threat to society both on the macro and micro scale as disinformation campaigns can stir up civil unrest and criminals leverage generative AI tools to fake kidnappings or commit fraud.
Integrating fact-checking tools into the generative AI system is one way of preventing misinformation, but educating users about the limitations and potential pitfalls of generative AI is equally critical.
Bias
Content produced through automated processes can sustain or magnify the biases present in the training data, leading to prejudiced, explicit, or aggressive language. This objectionable content necessitates human involvement to ensure alignment with the ethical standards of the employing organization.
For AI-focused companies, cultivating a team of diverse leaders and domain experts is crucial as these stakeholders will play a vital role in recognizing hidden biases in both data and models. Companies can also implement mechanisms to detect and address biases in both the training data and the generated content.
Copyright Infringements
Generative AI tools are trained using enormous datasets. When these tools generate text or produce code, their data source might remain unidentified meaning that generative AI may unintentionally infringe on copyrighted materials. In these instances, the potential for significant reputational and financial damage is high.
For example, pharmaceutical corporations that rely on intricate drug molecule formulations run the risk of developing a drug that is based on another company’s intellectual property. This is why human oversight and a firm understanding of the terms of use and licensing agreements for any data sources or content used during the training is so essential.
The rapid pace by which the generative AI market has grown has raised red flags for many experts. And fortunately, some are stepping up to spearhead a standard for generative AI ethics through the development of organizations like the AI Ethics Lab.
Ultimately only time will tell if we let the horse out of the barn too soon – or if there’s still time to put safety measures in place.
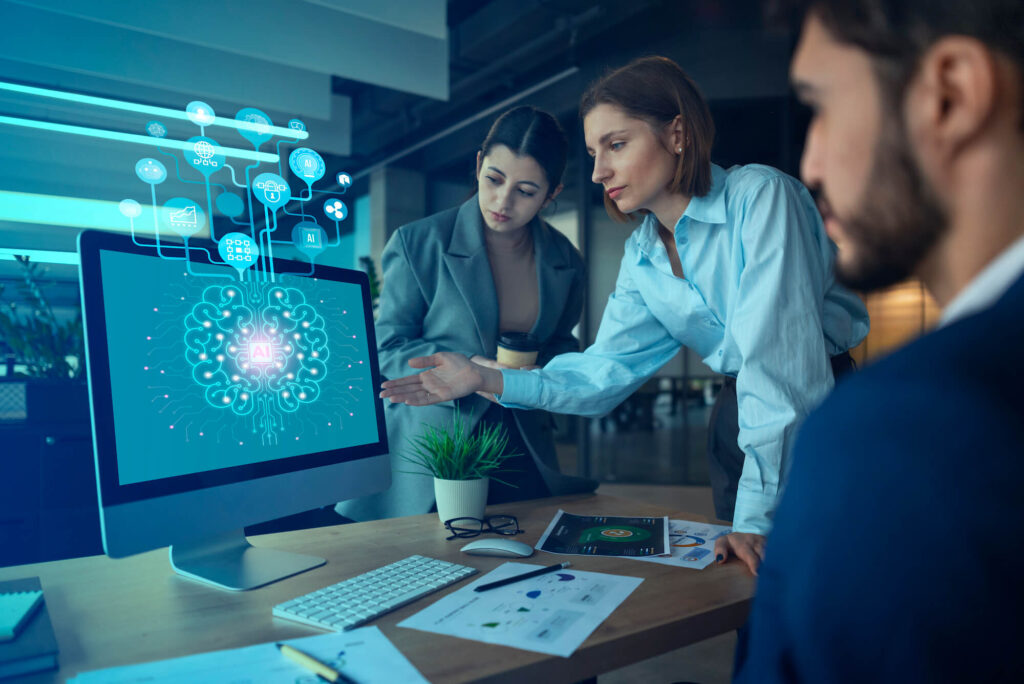
3 Trends to Watch in the Generative AI Market
1. Open-Source is On the Rise
With the release of several large language models (LLMs), there’s been a big push for sharing technology in the AI field. This is largely thanks to the efforts of startups, groups, and experts who want to challenge the idea of keeping these models closed and private.
Building state-of-the-art LLMs requires lots of resources, both technological (in terms of powerful computers) and human (in terms of the expertise and specialized skills required to develop these models). This can make it hard for many groups to create these models from scratch. But even the ones who can are choosing not to keep them private. Instead, they’re offering their models through application programming interfaces (or APIs). This change has created a situation where open-source AI is trying to fill in the gap by making these models more accessible to everyone.
2. Prompt Engineering is Expanding – Not Shrinking – the Job Market
Prompt engineering simply means crafting input instructions for generative AI models like ChatGPT and Dall-E. These instructions help AI models produce accurate and precise outputs. The main goal is to guide these models with specific and relevant instructions to generate accurate, relevant results.
If the instructions are not well-designed, the outputs might be generic or completely off base. But with a well-crafted prompt, the AI model can create outputs that closely match the desired outcome. That’s why prompt engineering has emerged as an entirely new profession in the burgeoning generative AI market. Rather than replacing humans with AI, many enterprises recognize that investing in training their employees in these new skill sets will pay dividends in the long run.
4. Increasing efficiency in customer service with the help of Generative AI
One of the most obvious and organic applications for text-based generative AI is customer service. When combined with advanced text-to-speech technology, generative AI has the potential to take over the entire customer engagement process, creating natural conversations that are indistinguishable from those with human agents. As a result, almost all customer conversations can be automated, making the process more streamlined and efficient.
With generative AI, businesses can offer personalized responses to customers, creating a more engaging and positive experience. Unlike traditional chatbots, which are often scripted and lack natural language processing capabilities, AI-powered chatbots can quickly and accurately respond to complex customer inquiries and even escalate the conversation to human agents when necessary. While there are significant benefits to this technology, it is important to consider potential drawbacks, such as the risk of losing the human touch in customer interactions and security and privacy concerns.
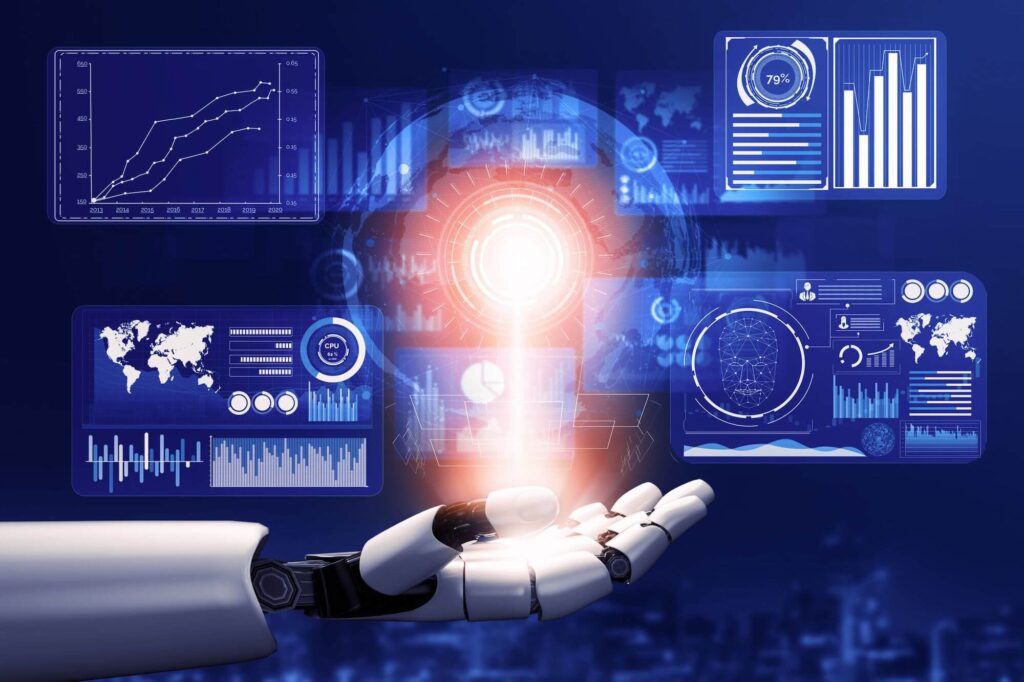
3 Challenges for Generative AI to Overcome
1. Future Challenges for LLMs
As LLMs evolve, the economic potential for generative AI looms large. However, LLMs have some logistical (as well as ethical) hurdles to overcome first. Experts highlight these as the major challenges LLMs face in the “AI arms race:”
- Hardware/chip shortage: Accessing GPU or TPUs will become more difficult, which is why some enterprises are looking to produce their own hardware, purpose-built for AI.
- Proprietary data: Models that can be trained with specific proprietary data will be in high demand.
- Data moat: Video generation models are already on the rise. Every actor in this market has access to public text-based material, so whoever unlocks the potential of video first will have a significant advantage.
- Cost: Applying LLMs on large collections of queries and text can prove to be costly. That’s why orchestration and other optimization methods are necessary.
- Instruction tuning: This will become increasingly important as AI requires explicit directions to deliver desirable and controlled outputs.
- Data sovereignty, privacy & regulations…more below.
2. Regulations Pump the Breaks on Generative AI’s Rapid Growth
The booming generative AI market has, understandably, spurred alarm in governments the world round. Likewise, the public has shown growing concern about AI’s potential social, economic, and security ramifications. Across the board, institutions agree that more legislation is needed for this technology to continue its evolution in a responsible, ethical manner.
In the US, the National Institute for Standards and Technology (NIST) put out an AI Risk Management Framework in January 2023, and the White House Office of Science and Technology Policy has published a blueprint for an AI Bill of Rights. The Senate’s guidelines are focused on transparency so that users can understand where LLM training data comes from, and how their data may be used. However, the US has historically demonstrated an “innovate first, regulate later” approach so the country is facing criticism for moving slowly.
Meanwhile, in the European Union, the AI Act has been in development for more than two years. Its purpose is to regulate the use of artificial intelligence in Europe by categorizing various AI tools based on their perceived level of risk, ranging from low to unacceptable. Depending on the level of risk, governments and companies using AI tools will have different responsibilities.
For example, high-risk AI systems, such as those used in critical infrastructures, employment, and law enforcement, will be subject to strict requirements and adequate risk assessment and mitigation systems. Limited and minimal or no-risk AI systems will also have specific transparency obligations and monitoring requirements. The legislation aims to remain future-proof and adaptable to technological change. It seeks to ensure that AI applications remain trustworthy, with ongoing quality and risk management by providers.
3. Cost of API Calls
Countless startups and other companies have started implementing generative AI into their products and services in an attempt to ride the tidal wave of enthusiasm for this tech and grab enterprises’ attention. However, if enterprises hope to adopt and integrate this technology, they will have to solve two looming problems:
- Cloud infrastructure costs: If using cloud-based services to deploy GPT-4 or any similar model, companies should consider the cost of cloud storage, computer resources, data transfer, and other cloud-related expenses. Cloud-based services give customers peace of mind that their data won’t feed into the main ChatGPT system. But this peace comes at a price: The same product could cost as much as 10 times what customers currently pay to use the regular version of ChatGPT.
- Transaction/API costs: AI providers may charge licensing fees or API usage costs for using their generative AI models. These costs can vary based on the number of API calls, the amount of data processed, and other factors.
Forging a Collective Future for Generative AI Ethics
In the grand tapestry of technological innovation, the opportunities presented by generative AI are as expansive as they are diverse. The road ahead holds the promise of remarkable successes that can reshape industries, enhance human experiences, and drive efficiency. However, it is equally true that the potential pitfalls are just as vast – misaligned incentives, ethical concerns, and unintended consequences loom on the horizon.
To navigate this intricate landscape, collaboration is essential. Researchers, developers, policymakers, and ethicists must synchronize their efforts to ensure that LLMs and generative AI technologies flourish in an environment of responsible and ethical integration. As these transformative tools find their way into healthcare, education, customer service, and creative content generation, it is the collective responsibility of the tech community to wield them for the betterment of society, mitigating risks and maximizing benefits. The possibilities are boundless, but the journey forward necessitates a united commitment to shaping the future of generative AI for the benefit of all.